FEATURED ARTICLES
This section includes brief descriptions of articles soon to be or recently published by the Journal of Mechanical Design. These featured articles highlight recent research developments and emerging trends in mechanical design.
For Abstracts and Full Articles please see ASME’s Digital Collection.
2024
September 29, 2024
Product innovations, from cell phones to commercial jet airliners, follow a pattern. Initially, firms compete with quite different designs. Eventually, some combination of form, features, and technologies become preferred and widely accepted as the dominant design, pushing alternate designs out of the market and changing the basis of product design competition. This paper explains the development of the dominant functional architecture that both underpins and precedes a dominant design. A dominant functional architecture refers to the specific technologies and technical know-how behind a dominant design. Based upon key innovations in sewing machines from the 1800s to the present time, the paper shows how to find recurrent functions across successive generations of designs as the basis for detecting the convergence toward a dominant functional architecture. The research findings have strong relevance to products such as autonomous vehicles and legged robots, where a dominant design has yet to appear. It is in the interest of firms to pay attention to convergence toward a dominant functional architecture even if physical designs appear divergent and can mislead firms into believing that a dominant design is a long way off. Tweet. When and what will be the dominant design for radical innovations such as autonomous vehicles or legged robots? This article recommends monitoring for the emergence of a dominant functional architecture even if physically observable designs look different.
2024
September 16, 2024
A major challenge in engineering analysis and design is to maintain consistency across models of the same engineered system at multiple levels of abstraction. For example, mechanical assemblies are commonly modeled at system-level (e.g., lumped-parameter networks of components) and component-level (3D models with detailed geometry and materials), whose comparison is nontrivial due to different representations and semantics. We present a novel, simulation-free approach to quantify consistency across a subset of such models; namely, linear time-invariant mass-spring-damper networks and corresponding elastic 3D objects deforming under mechanical loads. Their behavior is described by a finite vector of temporal signals, governed by systems of ordinary differential equations (ODEs), and spatio-temporal fields, governed by partial differential equations (PDEs), respectively. A major benefit of our approach is its ability to perform a priori consistency analysis rapidly (e.g., 5x faster than a posteriori comparison after solving ODEs and PDEs for state space dimensions larger than 30,000) even as the model complexity increases (e.g., only 2.2x slower when the number of equations is doubled). Our approach is validated through several mechanical design analyses, demonstrating its effectiveness in bridging the gap between different modeling abstractions and facilitating the integration between system-level and geometric designs.
2024
September 5, 2024
Unmanned systems have gained immense popularity due to their ability to carry out complex operations without human intervention. Imagine a ship that can dynamically adjust engine conditions to get just a little bit farther on its remaining fuel – spelling the difference between mission success and heading back to port early – that is the goal for these complex systems. The operation of these systems is heavily dependent on many of their subsystems and components that need to work together reliably. This article contributes to a new and general framework for operational planning of unmanned systems. Embedded in the framework are several techniques such as deep learning for predicting subsystem health state, dynamic Bayesian networks for system reliability analysis, and multi-objective optimization for reliability-based design of operational profiles. An application of the framework is demonstrated with a case study in engine cooling and control system for an unmanned surface vessel. For this case study, the operational profiles along a trade-off frontier are obtained while expected vessel speed and system reliability are maximized for the vessel mission.
Click on the link below to read entire article
A Reliability-Based Optimization Framework for Planning Operational Profiles for Unmanned Systems | J. Mech. Des. | ASME Digital Collection
2024
August 4, 2024
Design Theory
Theory And Methodology
Theory And Methodology
2024
May 3, 2024
While advances have been made in studying engineering design learning in the classroom, to date, such advances have not addressed hands-on, real-world learning experiences in university makerspaces. This article explores how such spaces support women engineers as designers, learners, makers, and community members.
Design Theory
Theory And Methodology
Theory And Methodology
2024
April 12, 2024
This article introduces an innovative design automation method aimed at systematically exploring and categorizing various 3D spatial layouts within interconnected engineering systems, encompassing diverse applications such as automotive cooling systems and building HVAC designs.
Design Automation
Design Optimization
Design Optimization
2024
April 12, 2024
In this study, we develop a cutting-edge method using graph neural networks to support the design decision-making of shared mobility systems, like bike-sharing programs.
Design Automation
Neural Networks
Neural Networks
2023
November 27, 2023
This research presents an AI approach to designing linkage systems, which are crucial in engineering for transforming rotational movement into precise periodic paths.
Design Automation
Design of Mechanisms and Robotic Systems
Machine Learning
Optimization
Design of Mechanisms and Robotic Systems
Machine Learning
Optimization
2023
November 27, 2023
This study examines how diverse initialization, such as Latin Hypercube Sampling (LHS) or Space-Filling Designs, impacts Bayesian Optimization (BO), one of the most widely used gradient-free optimizers.
Design Automation
Machine Learning
Machine Learning
2023
October 3, 2023
Design Automation
Topology Optimization
Topology Optimization
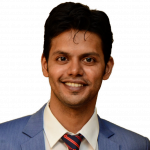
Keyword Search
Search by Year
Search by Topics