Yigitcan Comlek, Liwei Wang, Wei Chen
J. Mech. Des. May 2024, 146(5): 051706 https://doi.org/10.1115/1.4064133
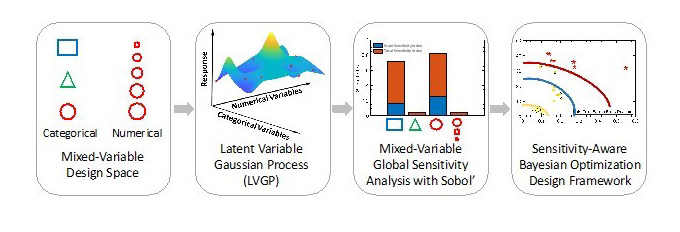
The flowchart of the mixed-variable global sensitivity analysis combined with the sensitivity-aware Bayesian optimization design framework
Global Sensitivity Analysis (GSA) is a powerful tool in engineering design to gain insights on how different inputs impact the outputs of a model. Traditionally, GSA has focused primarily on quantitative (numerical) variables. However, many engineering systems include, if not only, qualitative (categorical) variables such as material compositions and manufacturing methods, making the input-output relations even more complicated. In this work, the authors propose the first metamodel-based mixed-variable GSA method, by integrating Latent Variable Gaussian Process (LVGP) with Sobol’ analysis. LVGP uncovers correlations between categorical variables during machine learning, and a discrete Sobol sampling built on this model quantifies the contributions of mixed variables in GSA. This method was applied to metal-organic framework materials to understand how different factors influence their gas absorption capabilities. These insights were further integrated with multi-objective Bayesian optimization to create a sensitivity-aware design framework, achieving significant acceleration in exploring the Pareto front across many-level combinatorial design spaces. This method can be applied broadly to help engineers build accurate machine learning models, identify influential design variables, quantify input and output uncertainties, calibrate parameters, and achieve more efficient designs.