Eesh Kamrah, Seyede Fatemeh Ghoreishi, Zijian “Jason” Ding, Joel Chan, Mark Fuge
J. Mech. Des. Nov 2023, 145(11): 111703
https://doi.org/10.1115/1.4063006
This study examines how diverse initialization, such as Latin Hypercube Sampling (LHS) or Space-Filling Designs, impacts Bayesian Optimization (BO), one of the most widely used gradient-free optimizers. Common practice is to always recommend starting with diverse initial samples, but we show, to the contrary, that doing so has a critical downside. Non-diverse samples can speed up model hyper-parameter convergence (a model-building advantage), while diverse samples enhance function exploration (a Space Exploration advantage). Both advantages benefit BO differently, with initial sample diversity directly influencing this balance. Fixing BO hyper-parameters removes the Model Building advantage, making diverse samples consistently superior, but this is not true when, e.g., optimizing the kernel. Our findings caution against the current practice of universally using space-filling initializations, such as LHS and others, in BO, especially when you plan to optimize the BO hyper-parameters.
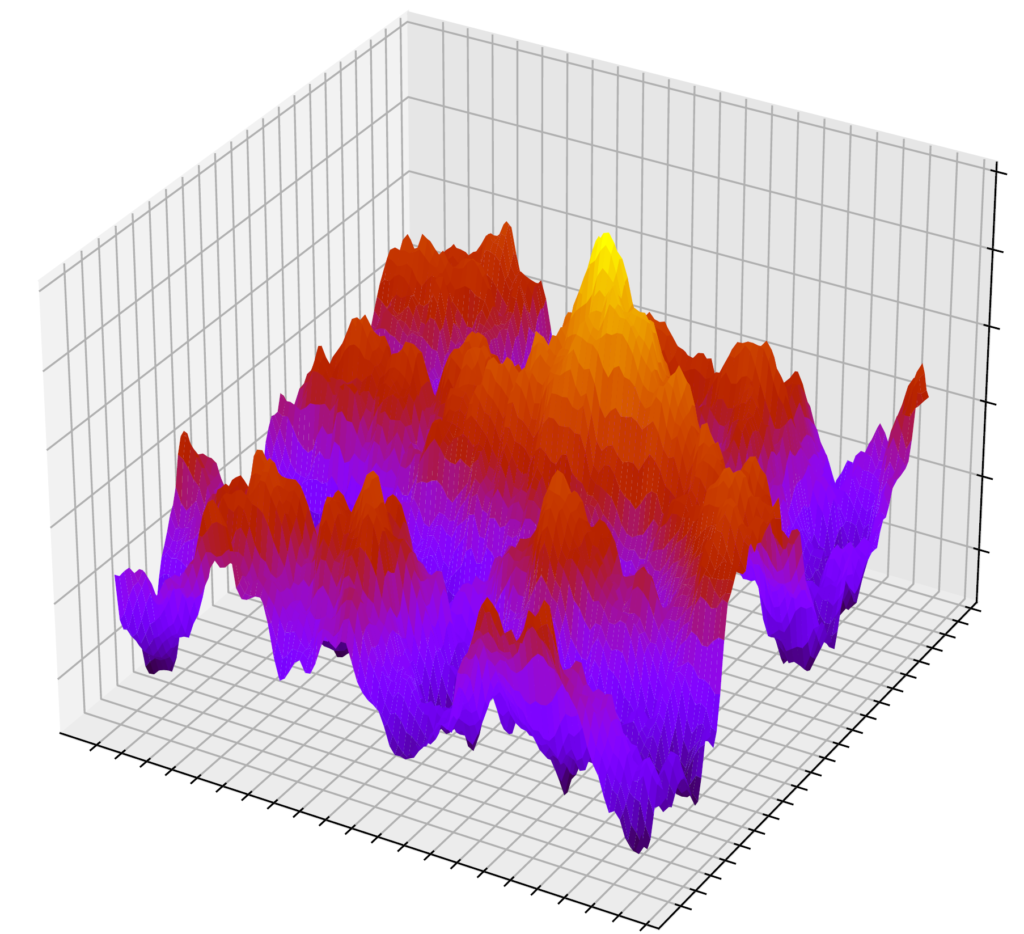