Mitchell B. Fogelson, Conrad Tucker, Jonathan Cagan
J. Mech. Des. Jul 2023, 145(7): 073303
https://doi.org/10.1115/1.4062147
This research presents an AI approach to designing linkage systems, which are crucial in engineering for transforming rotational movement into precise periodic paths. Traditional design methods face challenges due to the intricate relationship between a linkage’s structure and its geometry, both of which must be optimized to achieve the desired motion. This AI uses a single graph expansion rule to generate and evaluate linkage configurations rapidly. This AI utilizes a deep reinforcement learning agent that enhances the rule selection process to generate linkage designs. This process aims to align the output motion closely with a predefined goal while adhering to necessary design constraints. A graph convolution embedding network is used to interpret linkage designs as inputs to the AI effectively. This technique retains structural information of the linkage and other critical attributes, ensuring the AI receives a strong representation of the design. With this approach, engineers can swiftly create diverse linkage designs that meet specific motion objectives and comply with various design constraints. This enhances the efficiency of generating linkages that can perform complex tasks across different industries.
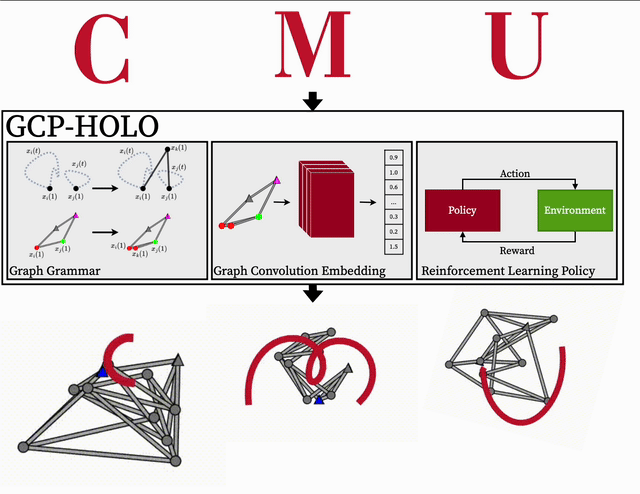