ASME. J. Mech. Des. September 2023; 145(9): 091702.
We present a new, neural network based topology optimization method that concurrently addresses three important aspects of design for additive manufacturing: (1) Automatically segmenting a monolithic part into smaller partitions to reduce print cost, (2) Optimizing the topology of each partition, and (3) Selecting the best printing direction orientation of each partition. This integration significantly minimizes support material usage. We train our model using 3D coordinates of the design domain, which then learns to produce a material density field and the associated partitioning of the density field through efficient gradient calculations. We demonstrate our method on compliance minimization problems with volume fraction constraints, adding support volume minimization as an objective. Our results show that this concurrent approach significantly decreases the requirement for support structures compared to traditional methods that optimize topology and print angle separately.
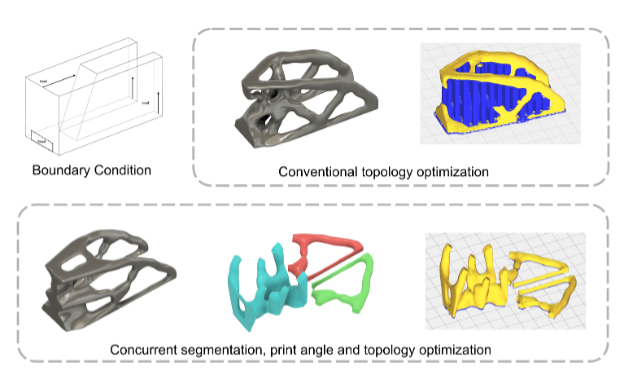