Ayush Raina, Jonathan Cagan, and Christoper McComb
ASME. J. Mech. Des. March 2023; 145(3): 031402
The ultimate goal for an autonomous design agent is the ability to learn and generalize how to solve design problems without a human’s help. We introduce a self-learning agent framework that integrates deep learning with tree search to achieve this goal. The tree search enables the agent to explore the problem space, and the deep policy network leverages self-generated experience to guide this search further. This paper introduces a sampling guided tree search and policy representation method that adapts the agent to arbitrarily complex action spaces that are often found in design. These agents discover high performing design strategies from scratch and further solve unseen boundary conditions without retraining. This work also illustrates the versatility of the framework by solving two distinct engineering design problems. Overall, this paper presents a methodology to self-learn high-performing and generalizable problem-solving behavior in an arbitrary problem space, circumventing the need for expert data, existing solutions, and problem-specific learning.
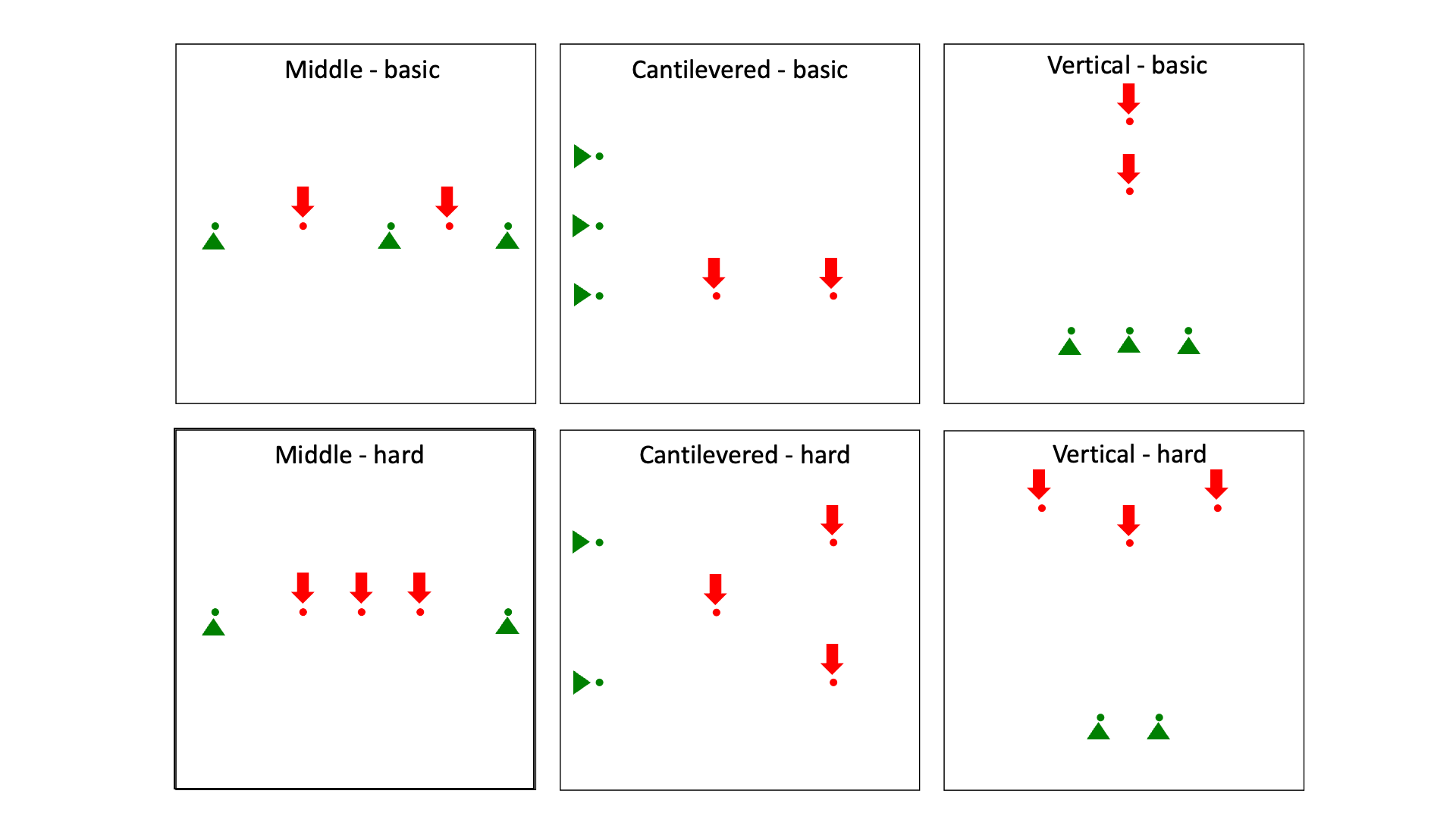