Jiaxin Wu and Pingfeng Wang, ASME. J. Mech. Des. March 2023; 145(3): 031705. https://doi.org/10.1115/1.4056078
In our modern world, complex systems such as power grids, transportation networks, and communication systems can be disrupted by both internal uncertainties and external factors like natural disasters. To make these systems more resilient, researchers have been working on design strategies that improve both reliability and recovery capabilities. However, designing robust systems is challenging because of the large scale and physical constraints of current interconnected systems. Existing methods need to simplify the design problem either by decreasing the size of the problem or making assumptions about the system’s physical properties to have a viable result. To tackle these issues, we developed a generative design method inspired by generative machine learning models that is suitable for versatile, large-scale systems. This framework includes a performance estimator and a candidate design generator that efficiently create new designs to mitigate potential failures and disruptions. The estimator can quickly predict the performance of these designs, enabling fast iterative learning, while the generator autonomously mines good design properties and outputs candidates with refined performance. We tested the method using synthetic supply chain networks and complex IEEE test power systems, demonstrating its effectiveness in designing resilient interconnected network systems.
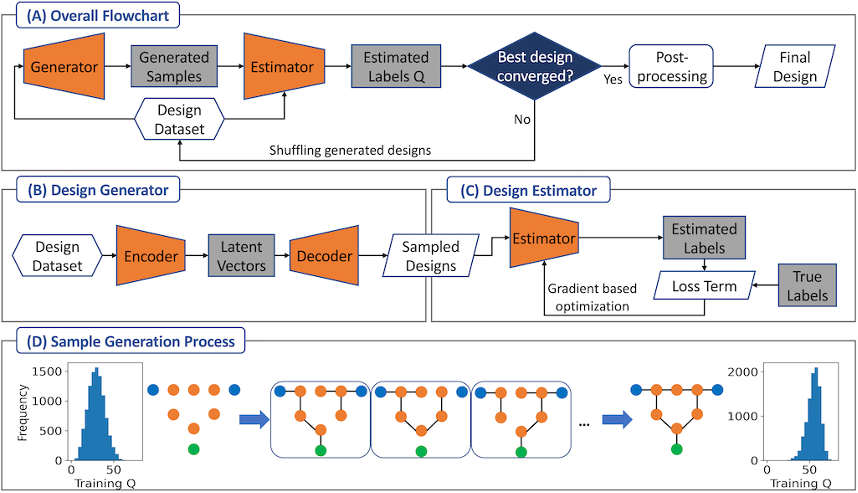
(A) The overall framework contains three parts—design generator, estimator, and post-processing for resilience; (B) the generator is a graph variational autoencoder (GVAE) model; (C) the estimator uses the graph convolutional network (GCN) to predict the performance of candidates; (D) through an iterative learning process, the sequence of design candidates approaches to the region that contains optimal designs.