Merel van Diepen and Kristina Shea
J. Mech. Des. Aug 2022, 144(8): 083305 (13 pages)
How does one decide on the gait of a soft robot, e.g. walk, jump or roll, and then the morphology and actuation to achieve this? Should we always look to nature for inspiration or can computers help us explore alternative designs? The longstanding challenge to co-design the morphology and actuation of soft robots makes designing them often a tedious, trial-and-error process. This paper proposes a Computational Design Synthesis (CDS) method for the automated design of virtual, soft robot morphologies with the automated co-design of actuation. In the first method, the actuation of designs is described by Parametric Actuation Curves (PACs) that model feedforward actuation patterns. For every morphology in the design process, a set of PACs is optimized that assumes symmetric and cyclic gaits. The second method, Soft Actor-Critic (SAC) reinforcement learning, removes this assumption as well as models feedback control, for comparison. PAC optimization within the CDS method results in a wide range of designs exhibiting walk, jump and roll gaits and is shown to improve the performance of designs with less computation. SAC is, however, deemed less effective, due to the need for design specific problem tuning for each new morphology. The SAC experiments do show that the best found soft robot gaits are symmetric and cyclic, thus verifying the assumptions made in the PAC formulation.
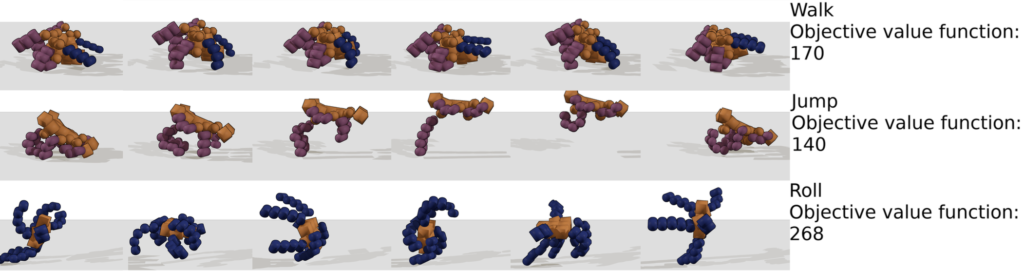
Three soft robot designs exhibit walking, jumping and rolling gaits that result from the automated co-design of morphology and actuation.